
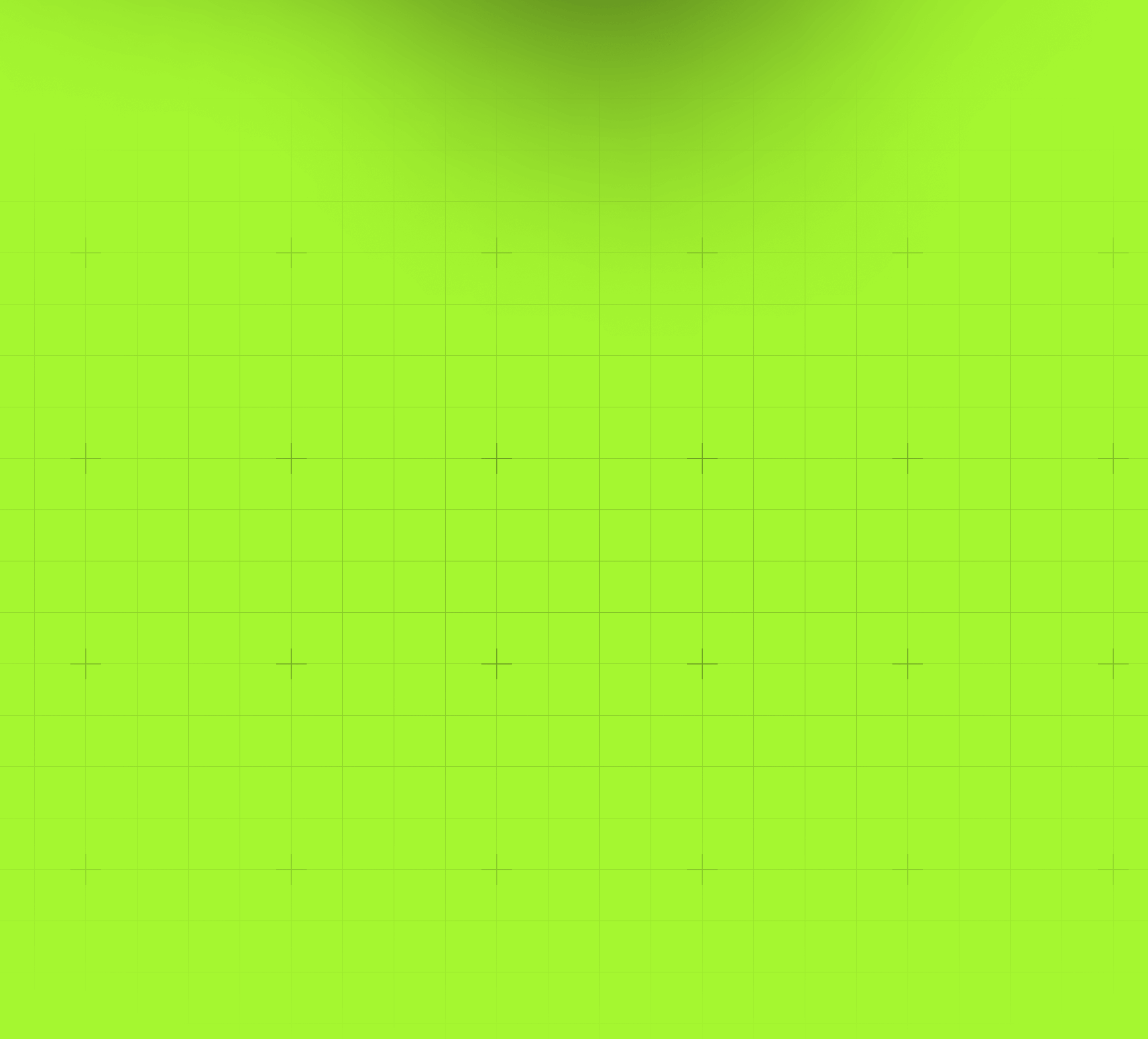
How AI is reshaping programmatic advertising
Programmatic advertising has become the dominant way to buy digital ads – in fact, an estimated 91% of all US digital display ad spending in 2023 flowed through programmatic channels roirevolution.com.
At this scale, no human team could manually manage the millions of ad impressions and split-second auctions occurring each day. Modern ad platforms increasingly rely on AI and machine learning to handle these real-time decisions and optimizations. AI algorithms now automate tasks that once required human judgment, bidding on ad space and tweaking campaigns on the fly to maximize efficiency and return on ad spend grapeseedmedia.com.
For marketers, understanding how AI is transforming programmatic advertising is key to staying competitive. We explore five critical areas in two separate sections below. From real-time bidding to personalization to fraud prevention. These are major areas where AI is revolutionizing programmatic buying, along with the opportunities and challenges it brings.
Automating ad buying and real-time bidding (RTB)
Programmatic advertising runs on real-time bidding, where ad inventory is bought and sold in milliseconds via automated auctions. AI has become the brain of this process. AI-powered RTB systems automatically place bids on ad impressions while analyzing vast data signals (like user behavior, context, and bid price) to decide the optimal bid instantly.
This level of automation ensures each impression is evaluated against predictive models – something impossible to do manually across billions of daily auctions. For example, machine-learning models can adjust bids dynamically based on historical patterns, user intent, and real-time conversion probabilities mediamint.com. In practice, this means the system might bid higher when a user is deemed likely to convert, and lower, or not at all, on less promising impressions.
These kinds of performance lifts demonstrate how AI can squeeze more value from each advertising dollar through intelligent bidding strategies. For marketers, AI-driven RTB means more efficient campaigns with better outcomes. This frees up human teams from micromanaging bids to focus on strategy and creative. Leveraging AI for programmatic buying is no longer optional; it’s an imperative to stay competitive
Smarter audience targeting
One of AI’s most game-changing impacts is how it refines audience targeting in programmatic campaigns. Traditionally, marketers defined broad segments to target. Now, AI can analyze vast datasets of user behavior to create granular audience profiles and segments that humans might miss. Sophisticated machine-learning algorithms sift through demographic data, browsing activity, purchase history, and more, to identify patterns, thereby allowing more accurate segmentation and ad targeting
In short, AI helps deliver the right ad to the right user at the right time with uncanny precision. Programmatic platforms such as PDi use predictive modeling to anticipate which users are most likely to convert as it adjusts targeting criteria in real time. This means campaigns continuously home in on high-value audiences as new data comes in.
One notable case study showed PDi’s AI Trading Desk drove a 40% lift in conversions compared to a human-operated trading desk.
For marketers, these capabilities mean higher ROI: more of your impressions turn into clicks or conversions when targeting is hyper-tailored to the individual.
Fraud detection and brand safety improvements
As programmatic advertising grew, so did the risks of ad fraud and brand safety. Fake clicks, bots, and sketchy websites can drain ad budgets and put brands in unwelcome contexts. AI is now on the front lines of defense in these areas. Digital ad fraud is a massive problem, an estimated $84 billion of ad spend was lost to fraud in 2023 alone (about 22% of global ad budgets), and that number could double to $170+ billion by 2028 adgully.com.
To combat this, AI-driven fraud detection tools monitor incoming ad traffic in real time and flag anomalies far more effectively than manual checks. Patterns of invalid traffic (bot networks, click farms, domain spoofing, etc.) can be identified through machine-learning models that recognize the subtle signals of fraudulent behavior.
When a suspicious activity spike or non-human pattern is detected, AI can automatically blacklist sources or filter them out of bidding, saving advertisers from wasting budget. Fraudsters typically follow the money in the industry, and as such, they continuously evolve their tactics. Luckily, AI gives us a fighting chance by reacting at machine speed.
Brand safety is the other side of this coin. Marketers want confidence that their ads won’t appear alongside inappropriate or harmful content. AI helps by scanning and understanding the context of web pages or videos before placing the ad. Natural language processing and computer vision algorithms can evaluate whether a page’s content aligns with brand safety guidelines. This goes beyond simple keyword blocklists; AI can assess nuances of context and sentiment. AI is also crucial in responding to new threats like generative AI content farms. Recent research showed hundreds of programmatic ads from major brands unknowingly ending up on low-quality websites filled with AI-generated text digiday.com.
These sites churn out clickbait articles, often with plagiarized or unreliable content, simply to earn ad revenue. AI can help detect such sites and exclude them from ad buys, thereby protecting brands from association with junk or fraudulent inventory. The goal for AI in brand safety is simple: filter out illegitimate traffic and unsafe content so that marketers’ budgets go toward real viewers on quality content, preserving both performance and brand integrity.
Performance optimization and predictive analytics
Driving better performance is at the heart of the value proposition of AI in programmatic advertising. Beyond automating bids and targeting, AI provides a continuous optimization engine that learns and improves campaigns in real time. These systems crunch enormous amounts of data on impressions, clicks, conversions, time of day, audience segments, and more, to find patterns that humans wouldn’t spot.
Predictive analytics is a key capability here. AI models can forecast how different factors will impact results and adjust strategies proactively. This might mean an AI model predicts which ad creative will perform best for a certain audience at a certain time, or which users are likely to make a purchase in the next week and will shift budget accordingly.
As the campaign runs, the AI continuously tests and iterates It allocates more spend to high-performing audience segments, pausing underperforming ads, and even tweaking frequency or pacing to hit KPI goals.
The impact of these AI optimizations can be significant on bottom-line metrics. Consider how AI-driven insights helped in real examples.
Amazon’s programmatic ad platform uses predictive analytics on shopping and browsing data to optimize ad placements, and this AI-powered placement strategy boosted advertisers’ ROI by 35% via zeroing in on users with high purchase intent and adjusting placements dynamically redresscompliance.com.
Similarly, Yahoo’s DSP applied big-data predictive models to refine audience segmentation and saw a 38% increase in ad engagement for B2B campaigns redresscompliance.com.
These examples illustrate how AI’s ability to anticipate outcomes and fine-tune campaigns can translate into better performance and efficiency. It’s not only about higher click or conversion rates either; AI can optimize for cost efficiency (e.g., lowering cost per acquisition by finding cheaper ad inventory that still converts), and for learning by running countless small experiments to continuously improve results.
From budget optimization, where algorithms push spend, to the best-performing channels or creatives in real time, adaptive frequency capping and timing, AI acts as a 24/7 campaign-optimization engine. The result for marketers is campaigns that get smarter over time, delivering stronger results without requiring constant manual intervention. Of course, human oversight is still crucial to set goals and interpret insights, but AI handles the heavy manual lifting to drive daily performance.
The ethical and data privacy challenges
While AI unlocks powerful capabilities in programmatic advertising, it also introduces new ethical and privacy considerations that marketers must navigate. Chief among these are concerns around consumer data privacy, potential algorithmic bias, and the transparency of AI-based decision making.
AI systems thrive on data. They often need to aggregate and analyze massive amounts of user information to target and personalize effectively. This raises flags if not managed responsibly. For example, AI-driven ad platforms might inadvertently compromise consumer privacy if vast datasets are mishandled or used without proper consent cmswire.com.
Stricter regulations worldwide (GDPR, CCPA and others) now govern how data can be collected and used, and AI doesn’t get a free pass here. Marketers integrating AI must ensure compliance with data protection laws and be transparent with users about data usage. The impending phase-out of third-party cookies has already pushed advertisers toward privacy-friendly solutions. AI is helping fill the gap with techniques like federated learning or probabilistic modeling based on anonymous signals, but balancing personalization with respect for user consent is an ongoing challenge mediamint.com.
Another ethical concern is bias and fairness in algorithmic targeting. AI models learn from historical data, which can reflect biases, and if not carefully monitored, they might optimize in ways that unintentionally discriminate or exclude certain groups – for instance, showing housing or job ads only to a particular demographic because the training data had that skew cmswire.com.
This kind of biased decision-making not only impacts privacy concerns but can damage brand reputation. Companies are beginning to put AI-based ethical guidelines in place to regularly test their algorithms for bias and to ensure a level of fairness in who sees their ads.
Relatedly, the “black box” transparency problem is real: AI often works in opaque ways that make it hard for marketers to understand why certain targeting or bidding decisions were made. This lack of can erode trust.
It’s important for vendors to offer as much transparency as possible. For example, providing reports on which factors influenced AI-driven decisions, so that advertisers feel in control and can justify their practices if questioned.
In summary, programmatic marketers should approach AI with a sense of responsibility. Some key challenges to address include:
- Data privacy and consent: AI’s hunger for data must be balanced with robust privacy safeguards. Strong data protection measures (anonymization, encryption), and clear user consent, are essential as AI-driven personalization can otherwise run afoul of privacy laws.
- As one marketing CEO noted, responsible AI use is critical when handling consumer data – misuse can lead to hefty fines and a loss of consumer trust
cmswire.com.
.
- Bias and fairness: Ensuring the training data and algorithms are not perpetuating bias is crucial. Diverse data sets, regular audits, and restriction of sensitive attributes help prevent discriminatory outcomes.
- Building fairness into AI models not only is ethically right but also widens your reach by not arbitrarily excluding segments of your potential audience.
- Transparency and accountability: Marketers need visibility within AI systems. It’s important to work with partners who will explain their AI’s logic in plain terms and allow some levers of control.
- Internally, teams should establish accountability for AI outcomes and treat AI as a tool that still requires human guidance. If an AI recommendation doesn’t feel right from a brand or ethical standpoint, humans must be ready to override it. In the age of AI, maintaining human oversight and ethical standards is non-negotiable.
Conclusion: navigating an AI-powered programmatic future
AI is undeniably transforming programmatic advertising – largely for the better. It’s making media buying more efficient, targeting more precise, creative more relevant, and operations more secure against fraud. Marketers adopting AI-driven programmatic strategies are already reaping the rewards in performance gains and productivity. In many ways, AI has become the co-pilot for digital marketers, handling the heavy lifting of data analysis and optimization at scale.
The competitive edge now lies in how well marketers can leverage these tools. Those who pair AI’s capabilities with their own strategic creativity will be able to deliver highly personalized, impactful campaigns that resonate with audiences and maximize ROI.
Yet an AI-powered future doesn’t mean a “hands off” or “set it and forget it” approach. Success in this new era requires a thoughtful, proactive approach. Marketers must continuously train and tune their AI models with quality data, interpret the insights AI provides, and ensure that marketing objectives and brand values are reflected in the machine’s decisions.
Equally important is staying vigilant about the pitfalls. Guarding customer privacy, pushing for transparency from ad tech partners, and keeping potential bias in check. Programmatic advertising has always been about the marriage of automation and data-driven strategy; AI simply supercharges that marriage.
By understanding both the promise and the responsibilities that come with AI, marketers can confidently navigate the programmatic landscape ahead. In doing so, they’ll not only achieve better campaign results but also build trust with consumers in an era when automation is everywhere. The bottom line: AI is reinventing how programmatic advertising works, and savvy marketers are embracing it as a powerful ally in delivering more effective campaigns.